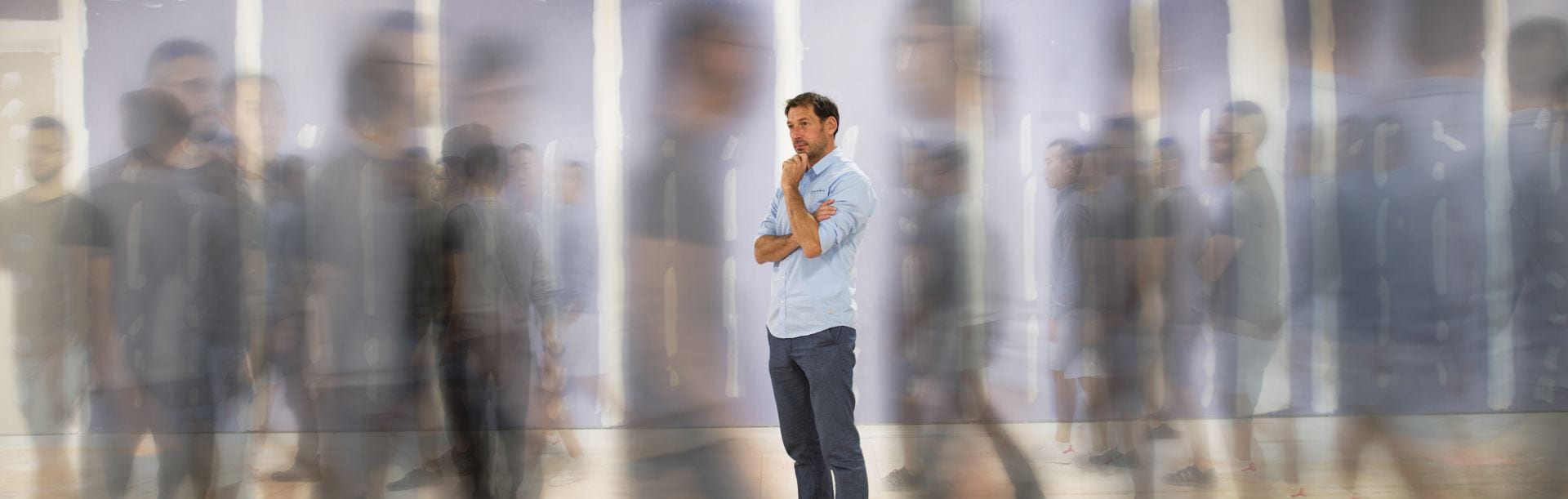
Past and present research
Most of our past work has been on characterizing the perceptual, cognitive, and neural basis of judgments from facial appearance. For a review of some of this work, see Todorov & Oh (2021). One of the important contributions of the lab was to introduce data-driven computational methods for visualizing the perceptual basis of social judgments from faces. These models capture systematic biases in judgments. See demonstrations for some of the resulting models of judgments. Recently, using generative adversarial networks we have built models capable of generating and manipulating the appearance of hyper-realistic faces. Currently, we are working on two interrelated lines of research: estimating idiosyncratic – specific to each individual – and shared contributions to face preferences and preferences in general and building models of idiosyncratic face preferences.
Present and future research I
How do stereotypes survive and are propagated even if they are not grounded in reality? How do these stereotypes lead to discrimination? When we think of bias and discrimination, we often think of direct discrimination, but much of discrimination is indirect. By choosing to interact with a “trustworthy-looking” partner, we can learn about their behavior, but not about the behavior of non-chosen partners. Moreover, these choices can systematically exclude non-preferred partners from mutually beneficial interactions. We are studying how our choices and features of the situation can lead to the confirmation and propagation of inaccurate stereotypes. Current studies explore how noisy information and prior biases combine to lead to stereotype confirmation and how observing the choices of others can amplify small biases in choices.
Present and future research II
Randomness is ubiquitous in life. Yet it is rarely directly observed and the role of chance events is often misinterpreted to fit a coherent narrative, whether the narrative is about personal or business success. We are studying the cognitive and experience-sampling mechanisms that can lead to systematic misinterpretations of random events and, specifically, to misattributions of luck to skill. We are developing experimental paradigms in which one’s success can be completely randomly determined or determined by a mixture of personal skills and randomness. Current studies explore how people experience and interpret consecutive lucky outcomes, whether being rewarded for luck changes the interpretation of these outcomes, and whether the ambiguity of the process generating the outcome contributes to misattributions of luck to skill.